Auctions, traffic, selfishness, and data privacy: It all comes down to math
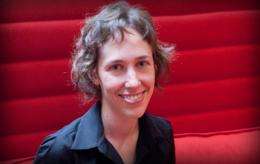
Every time you run a Google search, a split-second automated auction takes place to determine which of many competing companies will get to fill the ad space in your browsing window. The program controlling that auction is designed to fulfill a specific set of goals that probably differ from the goals of the individual companies. Similarly, your motivation during your morning commute is unlikely to be maintaining the overall flow of freeway traffic, and when you give a hospital your personal information, you're probably not trying to improve their data analysis.
These types of problems—where a conflict or tension exists between individual incentives and more global objectives—are Katrina Ligett's bread and butter. They fall into a category known as algorithmic game theory, and Ligett, a new assistant professor of economics and computer science at Caltech, uses ideas from computer science and mathematics to approach them.
"I'm interested in new algorithms, in understanding how difficult it is to solve problems," Ligett says. "Sometimes this comes from a particular application—maybe it's inspired by auctions that Google actually needs to run, for example."
Ligett says the field of algorithmic game theory has emerged in the last decade or so at the interface between computer science and economics. It got its start when computer scientists began to realize that they had something to offer to existing ideas in economics and game theory. For example, much of traditional game theory looks at equilibria, such as the Nash Equilibrium (developed by Nobel Prize–winning mathematician John Forbes Nash, Jr., and made famous on the big screen by A Beautiful Mind). That equilibrium describes a set of decisions that create a scenario in which no "players" want to change their decision, given how everyone else is making their decisions. "There is a huge amount of beautiful work in economics on such equilibria," Ligett says. "But there is relatively little work looking at things like how difficult it is to actually compute an equilibrium, and computer scientists have excellent tools to address such problems."
At first, computer scientists made up most of the field. But today, researchers are coming to algorithmic game theory from both directions—from computer science and from economics. Ligett says she was thrilled to come to Caltech in part because the Institute was looking for researchers to work specifically at this juncture. "There aren't that many places where computer scientists and economists actually talk to each other," Ligett says. "At Caltech, people are really interested in and committed to investigating at this intersection, and that's very appealing.
It wasn't always clear that Ligett would become a computer scientist. In high school and for a while afterward, she worked in an Army Corps of Engineers research lab in New Hampshire focused on studying the Arctic and Antarctic regions of the world. She got to see real scientists doing research and working in the field. She even got to travel to Barrow, Alaska, to study patterns of seasonal ice melt.
So when Ligett went to college at Brown University, she thought she'd go into a lab science—perhaps chemistry or physics. But that all changed when she took her first computer-science class. "I would get so wrapped up in problems that I was just really excited every week to work on my homework and projects for the class," she says. "So I thought, maybe I'll do a little bit more of this." Eventually, she switched over to computer science and mathematics, and went on to earn a PhD in computer science at Carnegie Mellon University.
Ligett's main research interests lie in trying to find better ways of thinking about and describing selfish behavior and in modeling alternatives to equilibrium states. "Some of my work says, 'What makes you think that people are going to end up at an equilibrium?'" she says. "Let's talk about where people might end up or what the whole system might look like if people act in a less prescribed way and just act selfishly. " She analyzes systems that never reach equilibrium and devises alternative models to try to account for situations where, for example, individuals might try to "game the system" and alter outcomes in unexpected ways.
In the area of data privacy, she's looking at the tension between privacy and the usefulness of data, trying to come up with new theorems to describe the relationship. Take, for example, a medical database, where Ligett might examine whether it is possible to mathematically transform the data in such a way that the database would provide researchers with meaningful data while still protecting the privacy of individuals.
Although the problems Ligett deals with involve complex, dynamic situations, most of what she does is good old paper-and-pencil math. "I might write down a mathematical description of people acting a certain way, using equations to establish the types of decisions people make. Once I have a mathematical model, I study the properties and consequences of the model," she says. So those equations on the white board in Ligett's new office? They might represent online auctions, the morning commute, or issues of data privacy. "I'm interested in the fundamental mathematics and in solving problems that are as general as possible," she says. "I hope that can be applied in a bunch of different ways."
Provided by California Institute of Technology